- Survey of Attack Graph Analysis Methods from the Perspective of Data and Knowledge Processing
- Two Formal Analyses of Attack Graphs
Attack Graph Models
- State-Enumeration - A graph-based system for network-vulnerability analysis (1998)
- Scenario - Automated generation and analysis of attack graphs (2002)
- NetSPA - Practical Attack Graph Generation for Network Defense (2006)
- MulVal - A Scalable Approach to Attack Graph Generation (2006)
- NetSPA - Practical Attack Graph Generation for Network Defense (2006)
- [Ours] TMG - Threat Modeling based on Graph Database (2022)
Summary from LAM-RAG
Method | Growth Rate in Hosts n | Are node hosts? | Is the graph acyclic? |
State-Enumeration | O(2^n) | Yes | No |
Scenario | O(2^n) | Partially | Yes |
MulVal | O(n^2) | No | Yes |
NetSPA | O(nlogn) | Sometimes | No |
TMG (Ours) | O(n^2) | Partially | Yes |
DeepHunter
Neiborhood Aggregation Strategy
- Soft similarity - GAN

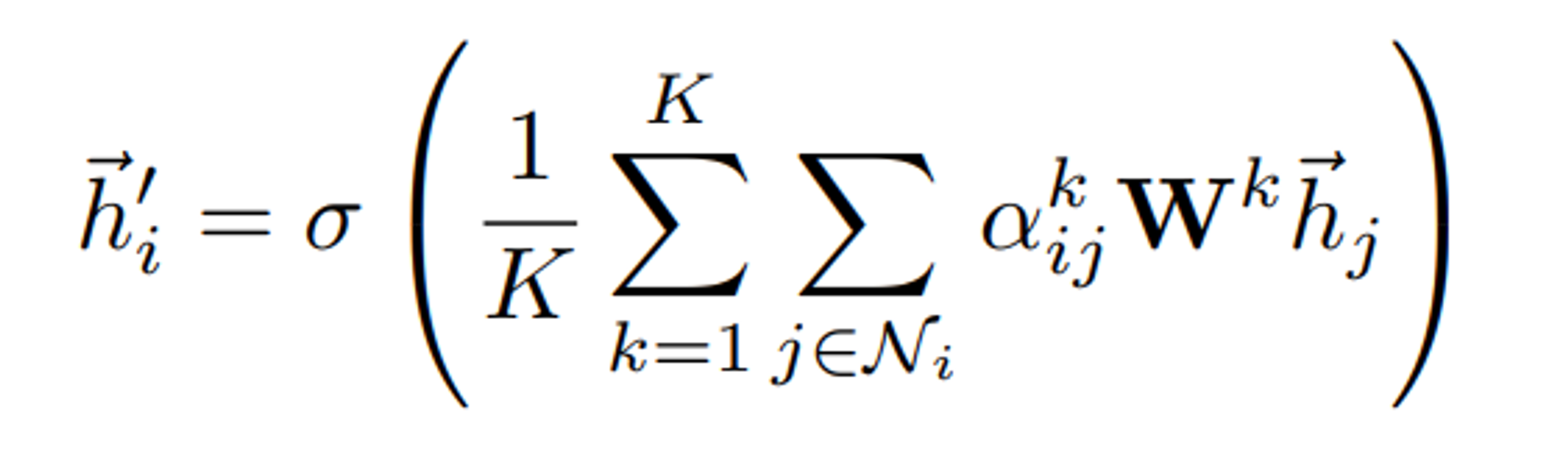
- Sampling different level of aggregated feature
Graph pre-training and fine-tuning
Multimodal
Meta-path
- GTN
- FastGTN
- Meta