Selected Key Researches based on LLM
Survey
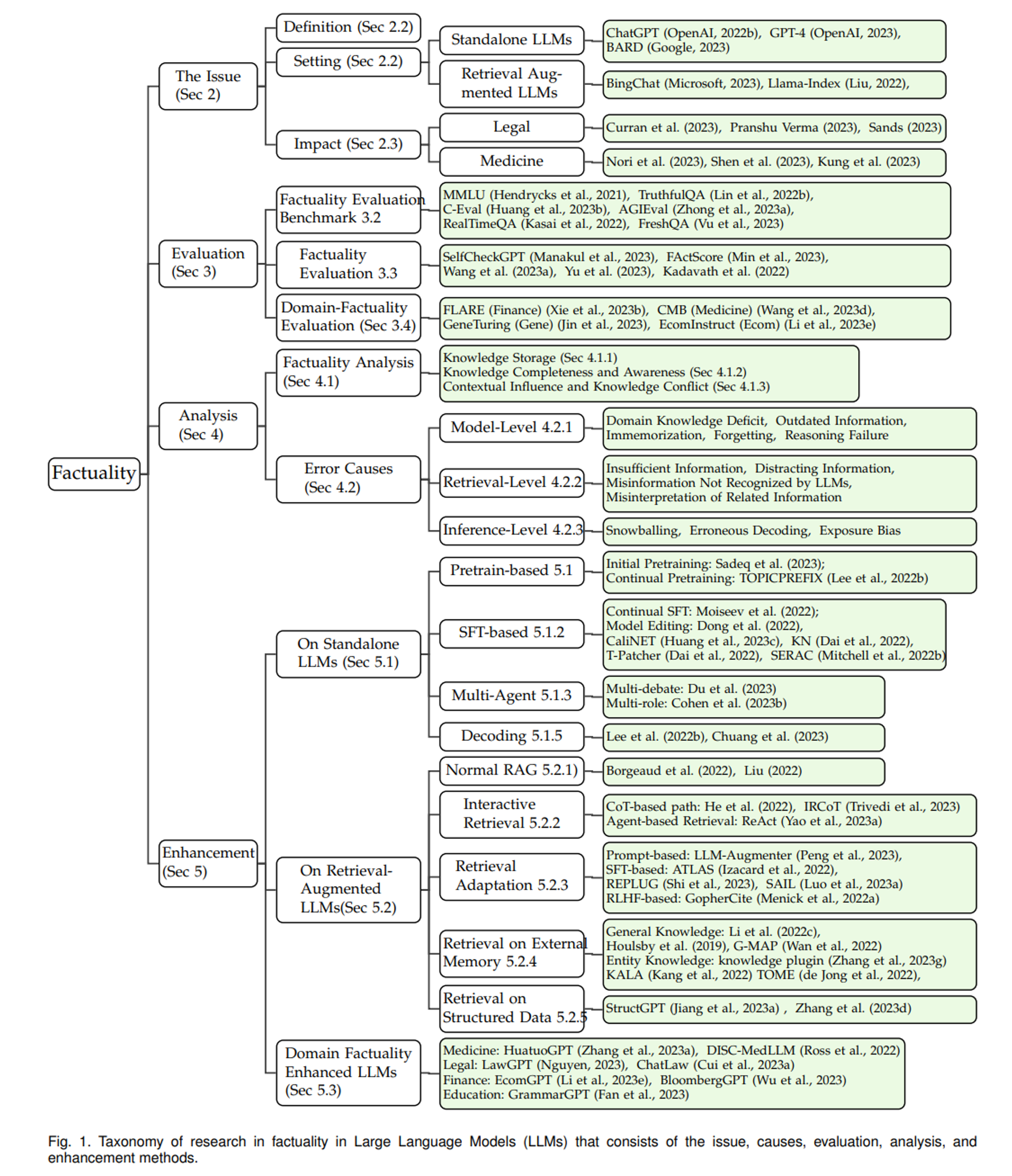
LLM family survey (LLM Summary)
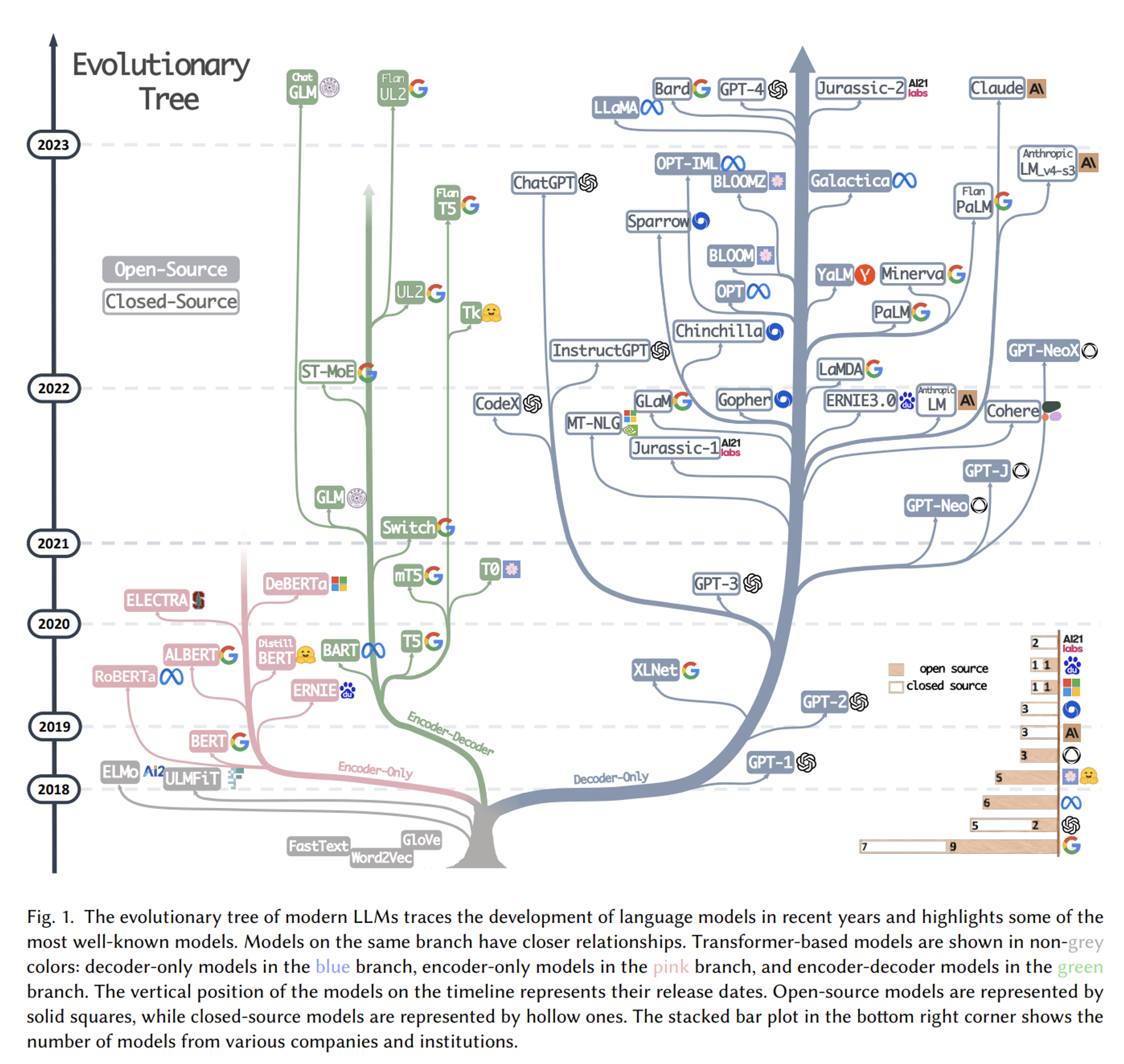
Tools:
PLMs
Colossal (based on LLAMA)
Falcon
LaMini-Flan-T5
Stable
Vicuna : 99% GPT-3.5
Dolly V2 (Pythia) : Business Lisence LLaMA
Factuality
CONNER COmpreheNsive kNowledge Evaluation fRamework
Papers - LLM Enhancement
[Text-to-SQL]
GNN to Transformer
[Multimodal] Single Diffuser for Video/Voice/Text/Image

[Model Structurel] RNN + Transfermer

[Multimodal] Output Keyboard/Mouse Opeartion Command (Recursive Criticism and Improvement (RCI))
[Multimodal] HuggingGPT: Solving AI Tasks with ChatGPT and its Friends in Hugging Face(JARVIS)
[Model Structurel] ‘My story has several episodes, kindly watch the ‘previously on’ (Amplify input length)
‘GPT-4 will judge you, rather than me.’ (LLM benchmark strategy)

‘You guys talk with each other’ (GAN-like LLM tuning)
‘Look at what you just said.’ (Key concept of AutoGPT family’)
‘You can join the exam many times’ (Self-consistency optimizing)
Backbones
[Interpretability]Elementary Proven of Relationships between Neurons in LLM and Knowlege Relationships (Selected Relationships in Knowlege Graph) by supressing or amplifying ‘knowledge neurons’ while observing Knowledge Attribution
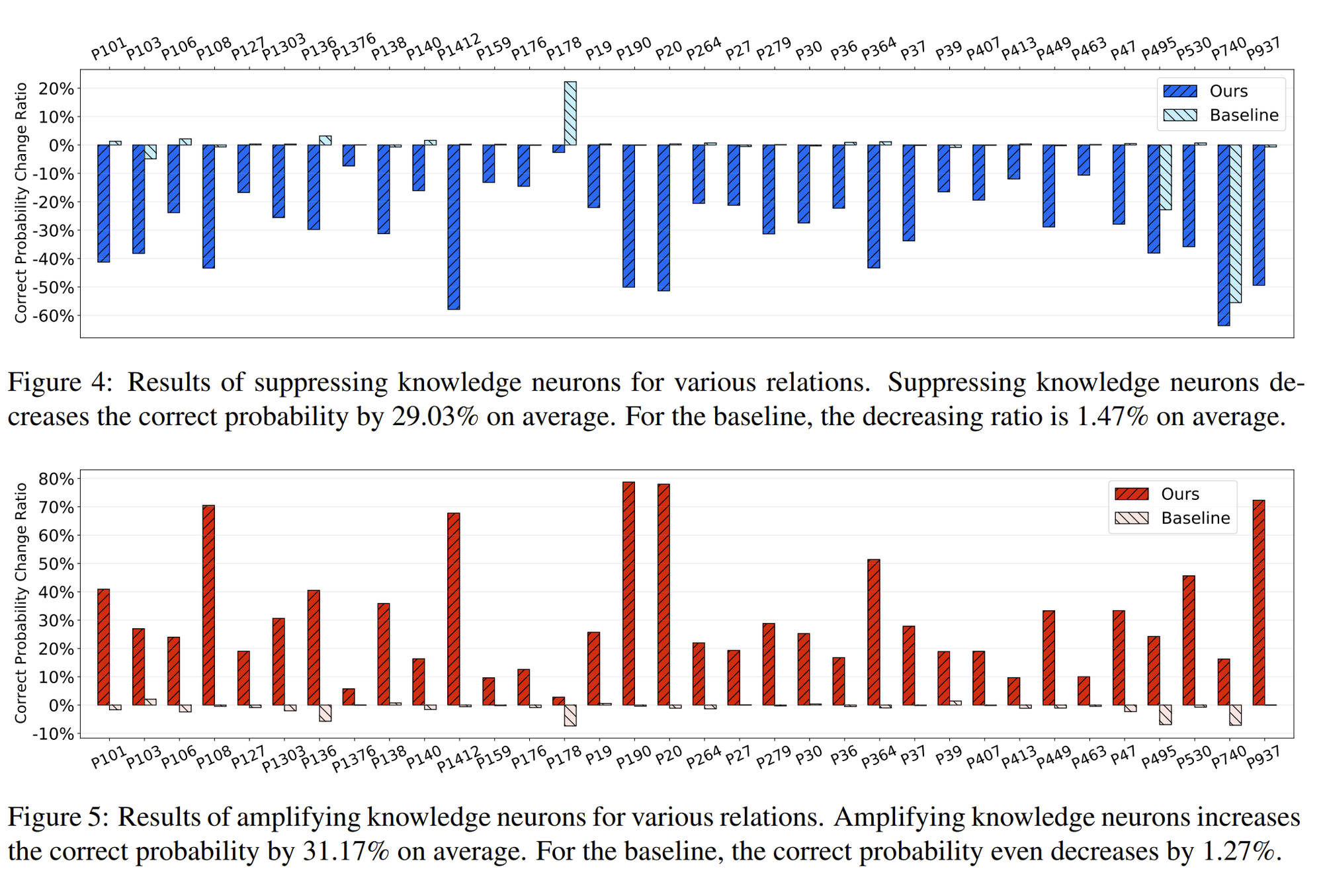
A new Gradient descent method
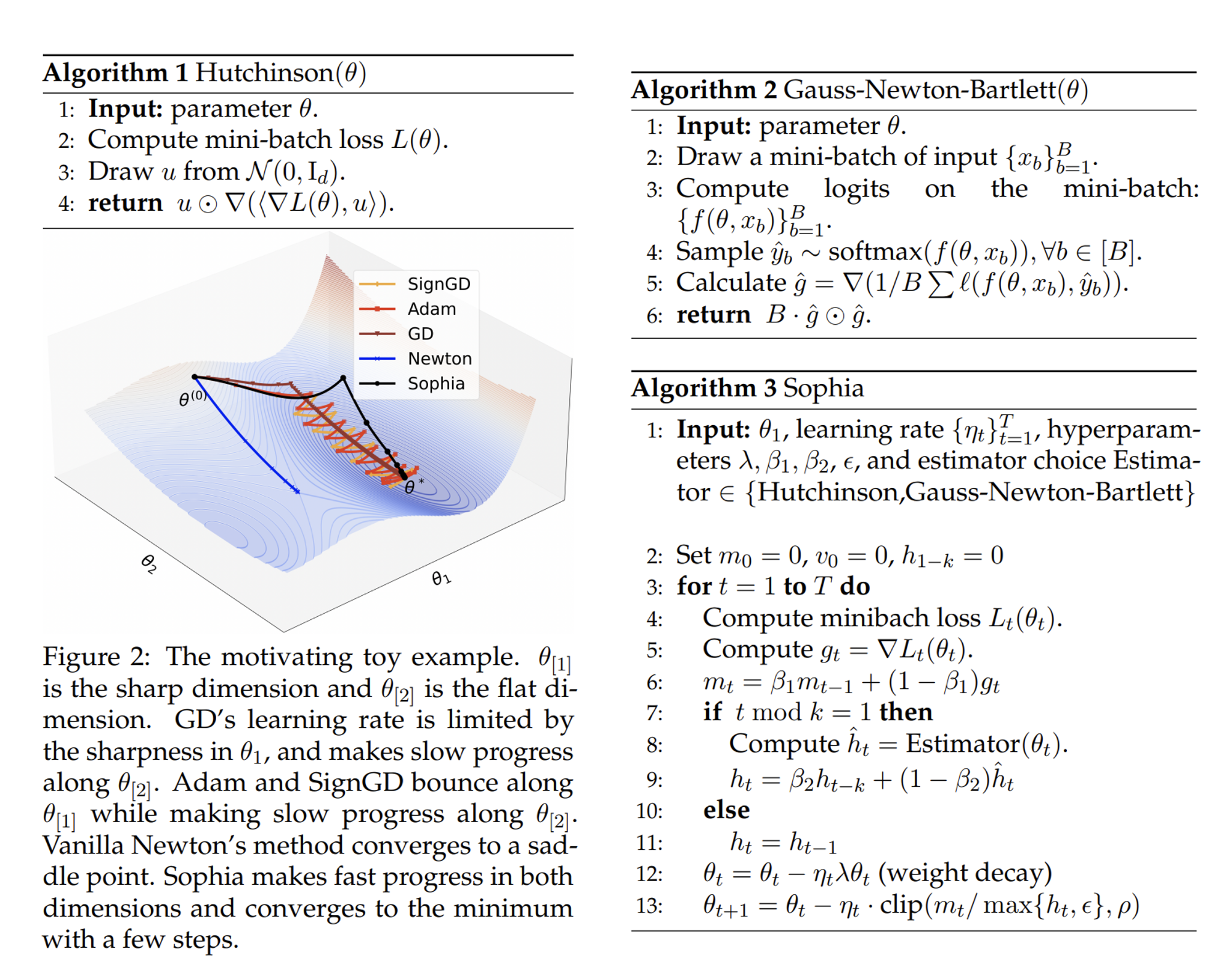
[Model Structurel]Soft Tuning
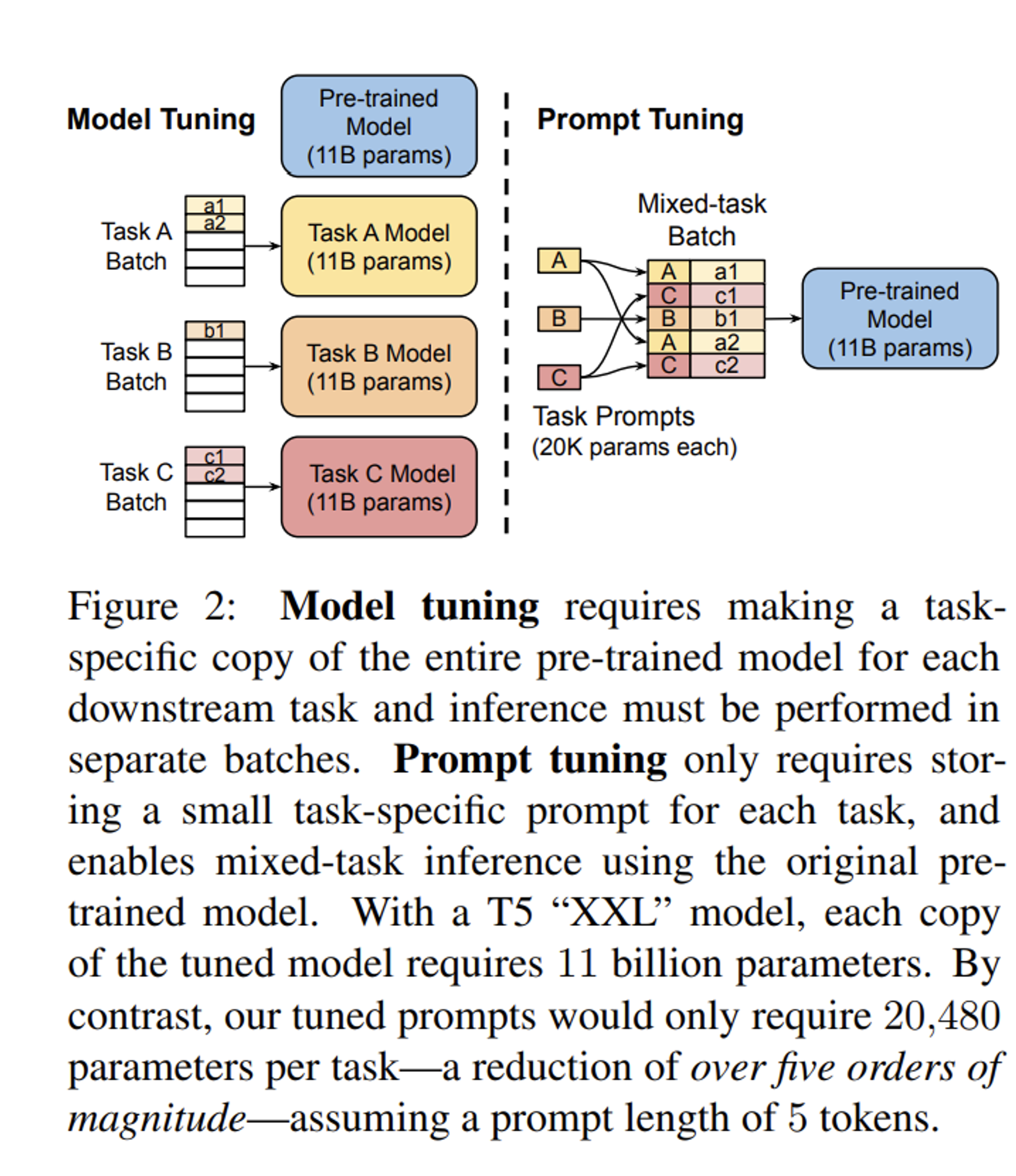
[Model Structurel]Attention
